The transformative potential of Large Language Models (LLMs) in technical support is capturing the attention of forward-thinking businesses. However, transitioning from pilot projects to full-scale adoption requires a compelling business case that resonates with decision-makers and clearly demonstrates the return on investment (ROI).
This blog post outlines a structured approach to building a data-driven business case for advanced LLM adoption in technical support, ensuring you secure buy-in and unlock the full potential of this game-changing technology.
I. Laying the Foundation: Understanding the Stakes and the Opportunity
Before presenting the solution, clearly articulate the challenges and opportunities that make LLM adoption compelling:
1. The Evolving Landscape of Technical Support:
Acknowledge the increasing pressure on support teams:
- Increasing Product Complexity: As technology evolves at an unprecedented pace, products and services are becoming more intricate, demanding a higher level of technical expertise from support agents.
- Rising Customer Expectations: Customers expect instant gratification, personalized support, and accurate solutions, regardless of the channel or time of day.
- Growing Support Volumes and Costs: Expanding customer bases and product complexity lead to higher ticket volumes, putting a strain on resources and budgets.
2. The Promise of Advanced LLMs:
Position LLMs as the answer to these challenges:
- Automating Repetitive Tasks: LLMs can handle a significant volume of routine inquiries, freeing human agents to focus on more complex issues requiring specialized expertise.
- Providing Real-Time Agent Assistance: LLMs can act as intelligent assistants, offering agents instant access to relevant knowledge bases, recommended solutions, and even code snippets, drastically reducing handle time and improving first-call resolution rates.
- Personalizing the Support Experience: LLMs can analyze customer data, past interactions, and product usage to deliver tailored solutions, proactive support, and personalized recommendations, enhancing customer satisfaction.
- Enhancing Knowledge Management: LLMs can analyze support interactions, identify content gaps, and even assist in generating knowledge base articles, ensuring customers and agents access the most relevant information.
II. Quantifying the Value: Building a Data-Driven Case for ROI
Moving beyond general benefits, quantify the impact of LLMs with data-driven insights:
1. Identify Key Performance Indicators (KPIs):
Focus on metrics directly aligned with business objectives:
- First-Contact Resolution Rate (FCR): Demonstrates improved efficiency and customer satisfaction by measuring the percentage of issues resolved on the first interaction.
- Average Handle Time (AHT): Tracks the average time agents spend resolving issues, showcasing increased productivity and cost savings.
- Customer Satisfaction (CSAT) and Net Promoter Score (NPS): Gauge customer sentiment and loyalty, highlighting improved support experiences and reduced churn.
- Support Cost per Ticket: Calculates the average cost of resolving a support ticket, demonstrating cost optimization achieved through automation and efficiency gains.
- Agent Productivity and Utilization: Measures the number of tickets resolved per agent and the time spent on value-added tasks, illustrating improved workforce utilization and agent satisfaction.
2. Establish a Baseline and Set Realistic Targets:
Gather historical data on your chosen KPIs to establish a clear baseline for comparison. Research industry benchmarks and set ambitious yet attainable targets for improvement post-LLM implementation.
3. Develop a Cost-Benefit Analysis:
Outline the costs associated with LLM adoption:
- Software Licensing and Implementation: Include expenses related to acquiring, customizing, and integrating the LLM solution with your existing infrastructure.
- Data Preparation and Training: Factor in the resources required for data collection, cleaning, annotation, and ongoing model training and fine-tuning.
- Ongoing Maintenance and Support: Account for costs associated with LLM maintenance, software updates, and ongoing technical support from the vendor.
4. Project the Return on Investment (ROI):
Combine projected KPI improvements with estimated costs to calculate the anticipated ROI over a defined timeframe (e.g., 12, 24, or 36 months). Present the ROI clearly and compellingly, highlighting short-term gains and long-term value creation.
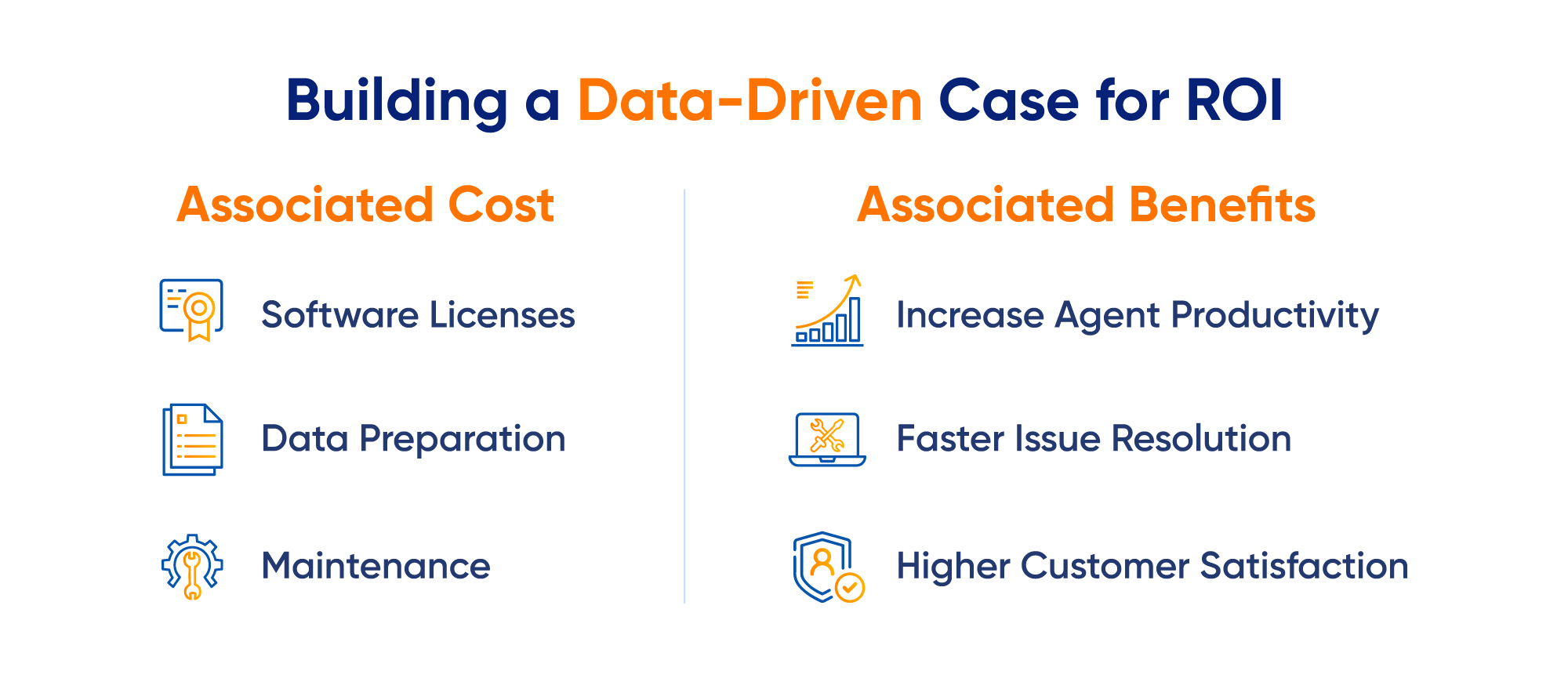
Early Successes with SearchUnify Customers:
At SearchUnify, we’ve witnessed firsthand the transformative power of LLMs in technical support. Our customers across various industries are experiencing significant improvements in key metrics:
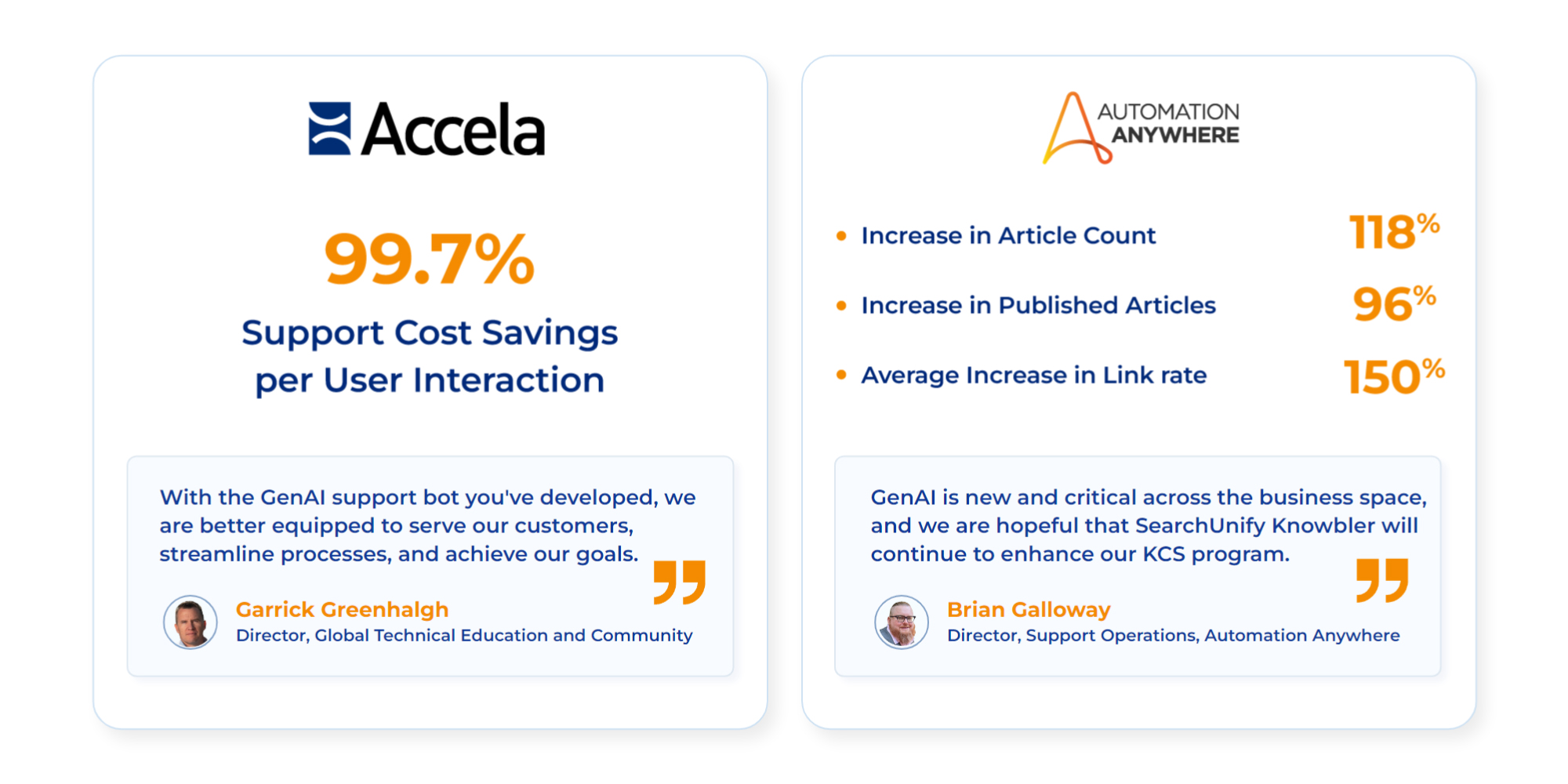
Conclusion:
Building a compelling business case for advanced LLM adoption in technical support requires a data-driven approach that quantifies the value proposition and addresses potential concerns. By focusing on measurable improvements in KPIs, outlining a clear ROI, and showcasing early successes, you can secure buy-in and pave the way for a transformative customer support experience.
Ready to explore how SearchUnify can help you unlock the power of LLMs in your technical support operations? Schedule a demo today!